Google Protobuf的介绍可以参考[附]的内容,这里介绍在Ubuntu14.04上编译安装指定版本的protobuf的操作步骤,这里以2.4.1为例:
1.Ubuntu14.04上默认安装的是2.5.0,默认安装到/usr/bin目录下,如下:
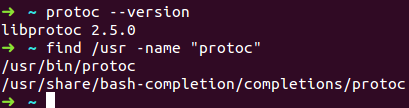
2.从 https://github.com/google/protobuf/releases?after=v3.0.0-alpha-1下载protobuf2.4.1源码 protobuf-2.4.1.zip;
3.将其存放到新建的protobuf目录下,通过unzip命令进行解压缩:
$ unzip protobuf-2.4.1.zip
4.解压缩后的文件会放到protobuf-2.4.1目录下,将终端定位到此目录内;
5.依次执行:
$ ./autogen.sh
$ ./configure
$ make
$ make check
$ sudo make install
$ sudo ldconfig # refresh shared library cache
有时make check会报gtest或gmock的错误,可以先不用管,直接执行make install即可。
6.默认2.4.1会安装到/usr/local/bin目录下,然后通过软链接的方式将新生成的protoc链接到/usr/bin即可,如下图:
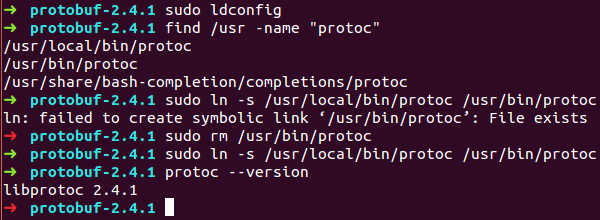
[附]
Google Protocol Buffers(简称Protobuf),是Google的一个开源项目,它是一种结构化数据存储格式,是Google公司内部的混合语言数据标准,是一个用来序列化(将对象的状态信息转换为可以存储或传输的形式的过程)结构化数据(即行数据,存储在数据库里,可以用二维表结构来逻辑表达实现的数据)的技术,支持多种语言诸如C++、Java以及Python。可以使用该技术来持久化数据(将内存中的数据模型转换为存储模型或者将存储模型转换为内存中的数据模型)或者序列化成网络传输的数据。它是语言无关、平台无关的、扩展性好的用于通讯协议、数据存储的结构化数据序列化方法。相比较一些其它的如XML技术而言,该技术的一个明显特点就是更加节省空间(以二进制流存储)、速度更快也更加灵活。
通常,编写一个Protobuf应用需要三步:
1.定义消息格式文件,最好以proto作为后缀名:proto文件即消息协议原型定义文件,在该文件中可以通过使用描述性语言来定义程序中需要用到的数据格式。
在Protobuf术语中,结构化数据被称为message。message是消息定义的关键字,等同于C++中的struct/class。在.proto文件中,message可以嵌套message。
在一个.proto文件中,可以用import关键字引入在其它.proto文件中定义的消息,这可以称作import message或者dependency message。import message的用处主要在于提供方便的代码管理机制,可以将一些公用的message定义在一个package中,然后在别的.proto文件中引入该package,进而使用其中的消息定义。
一般情况下,使用Protobuf会先写好.proto文件,再用Protobuf编译器生成目标语言所需要的源代码文件,然后将这些生成的代码和应用程序一起编译。在某些情况下,可能无法预先知道.proto文件,需要动态处理一些未知的.proto文件,这就需要动态编译.proto文件,并使用其中的message。Protobuf提供了google::protobuf::compiler包来完成动态编译的功能。
在定义Protobuf消息时,可以使用和C++代码同样的方式添加注释(“//”)。枚举值之间的分隔符是分号,而不是逗号。可以为枚举值指定任意整型值,而无需总是从0开始定义。可以在同一个.proto文件中定义多个message,这样便可以很容易的实现嵌套消息的定义。
每个字段(field)都有一个修饰符、字段类型和字段标签(Tag,数字1,2,…)组成。
三个修饰符(required/repeated/optional):
(1)、required:初值是必须要提供的,否则字段的值是未初始化的。在序列化和在反序列化(解析,从序列化的表示形式中提取数据,并直接设置对象状态)时对该字段的解析会失败。
(2)、optional:如果未进行初始化,那么一个默认值将赋予该字段,也可以自己指定默认值。
(3)、repeated:该字段可以重复多个,出现0次也是可以的。
一个格式良好的消息一定要含有一个required字段,表示该值是必须要设置的。每个消息中可以包含0个或多个optional类型的字段。repeated表示的字段可以包含0个或多个数据。
如果打算在原有消息协议中添加新的字段,同时还要保证老版本的程序能够正常读取或写入,那么对于新添加的字段必须是optional或repeated。
字段标签:消息中的每一个字段都有一个独一无二的数值类型的Tag,标示了字段在二进制流中存放的位置,这个是必须的,而且序列化与反序列化时相同的字段的标签值必须对应,否则反序列化时会出现意想不到的问题。
在.proto文件中定义消息的字段标签时,可以是不连续的,但是如果将其定义为连续递增的数值,将获得更好的编码和解码性能。
Tag用来在消息的二进制格式中识别各个字段的,一旦开始使用就不能够再改变。最小的标签号可以从1开始,最大到2^29-1.不可以使用其中的19000-19999的标识号,Protobuf实现中对这些进行了预留。如果非要在.proto文件中使用这些预留标识号,编译时就会报警。
如果字段的属性值是固定的几个值,可以使用枚举。
2.使用Google提供的Protobuf编译器使proto文件生成代码文件,针对C++语言,一般为.pb.h和.pb.cc文件,主要是对消息格式以特定的语言方式描述。
3.将生成的.pb.h和.pb.cc文件加入自己的工程中,使用Protobuf库提供的API来编写应用程序。
Protobuf支持的标量数值类型:double、float、int32、int64、uint32、uint64、sint32、sint64、fixed32、fixed64、sfixed32、sfixed64、bool、string、bytes。
Protobuf消息更新原则:(1)、不要修改已经存在字段的标签号;(2)、任何新添加的字段必须是optional和repeated限定符,否则无法保证新老程序在互相传递消息时的消息兼容性;(3)、在原有的消息中,不能移除已经存在的required字段,optional和repeated类型的字段可以被移除,但是它们之前使用的标签号必须被保留,不能被新的字段重用;(4)、int32、uint32、int64、uint64和bool等类型之间是兼容的,sint32和sint64是兼容的,string和bytes是兼容的,fixed32和sfixed32,以及fixed64和sfixed64之间是兼容的,这意味着如果想修改原有字段的类型时,为了保证兼容性,只能将其修改为与其原有类型兼容的类型,否则就将打破新老消息格式的兼容性;(5)、optional和repeated限定符也是相互兼容的;(6)、不需要的字段可以删除,删除字段的Tag不应该在新的消息定义中使用;(7)、不需要的字段可以转换为扩展,反之亦然,只要类型和数值依然保留。
在.proto文件中定义的包名,该包名在生成对应的C++文件时,将被替换为名字空间名称。
Protobuf允许在.proto文件中定义一些常用的选项,这样可以指示Protobuf编译器帮助我们生成更为匹配的目标语言代码。Protobuf内置的选项被分为三个级别:(1)、文件级别,这样的选项将影响当前文件中定义的所有消息和枚举;(2)、消息级别,这样的选项仅影响某个消息及其包含的所有字段;(3)、字段级别,这样的选项仅仅影响与其相关的字段。
optionoptimize_for = LITE_RUNTIME; optimize_for是文件级别的选项,Protobuf定义三种优化级别SPEED/CODE_SIZE/LITE_RUNTIME。缺省情况下是SPEED。(1)、SPEED:表示生成的代码运行效率高,但是由此生成的代码编译后会占用更多的空间;(2)、CODE_SIZE:和SPEED恰恰相反,代码运行效率较低,但是由此生成的代码编译后会占用更少的空间,通常用于资源有限的平台,如Mobile;(3)、LITE_RUNTIME:生成的代码执行效率高,同时生成代码编译后的所占用的空间也是非常少,这是以牺牲Protobuf提供的反射功能为代价的。因此在C++中链接Protobuf库时仅需链接libprotobuf-lite,而非libprotobuf.对于LITE_MESSAGE选项而言,其生成的代码均将继承自MessageLite,而非Message。MessageLite类是Message类的父类。
对于数值型的repeated字段,可通过添加[packed= true]的字段选项,以通知Protobuf在为该类型的消息对象编码时更加高效。如果设置该选项,那么元素数量为0的repeated字段将不会被编码,否则数组中的所有元素会被编码成一个单一的key/value形式。该编码形式,对包含较小的整型元素而言,优化后的编码结果可以节省更多的空间。该选项仅适用于2.3.0以上的Protobuf。
[default = default_value]:optional类型的字段,如果在序列化时没有被设置,或者是老版本的消息中根本不存在该字段,那么在反序列化该类型的消息时,optional的字段将被赋予类型相关的缺省值,如bool被设置为false,string默认为空串,数字类型默认0,枚举类型,默认为类型定义中的第一个值。.Protobuf也支持自定义的缺省值。
Protobuf中的消息都是由一系列的键值对构成的。每个消息的二进制版本都是使用标签号作为key,而每一个字段的名字和类型均是在解码的过程中根据目标类型(反序列化后的对象类型)进行配对的。在进行消息编码时,key/value被连接成字节流。在解码时,解析器可以直接跳过不识别的字段,这样就可以保证新老版本消息定义在新老程序之间的兼容性,从而有效地避免了使用older消息格式的older程序在解析newer程序发来的newer消息时,一旦遇到未知(新添加的)字段时而引发的解析和对象初始化的错误。
Protobuf目前有2个大版本,proto3是最新版本,它引入了一个新的语言版本Protocol Buffers,以及一些新特性在现有的语言版本proto2。proto3简化了Protocol Buffers语言,易用性更高使它可以成为一个广泛的编程语言。目前,proto3只有beta版,注意,两个语言版本的api不是完全兼容。
一些来自Google的工程师们指出使用required弊大于利,尽量使用optional和repeated。
Protobuf支持更深层次的嵌套和分组嵌套,但是为了结构清晰可见,不建议使用过深层次的嵌套。
Protobuf编译器会为每个消息生成一个类,每个类包含基本函数、消息实现、嵌套类型、访问器等部分。
由于一些历史原因,基本数值类型的repeated的字段并没有被尽可能的高效编码。在新的代码中,用户应该使用特殊选项[packed = true]来保证更高效的编码。
enum值是使用可变编码方式的,对负数不够高效,因此不推荐在enum中使用负数。
通过扩展,可以将一个范围内的字段标签号声明为可被第三方扩展所用。然后,其他人就可以在自己的.proto文件中为该消息类型声明新的字段,而不必去编辑原始文件了。关键字为”extensions”
如果你的消息中有很多可选字段,并且同时至多一个字段会被设置,你可以加强这个行为,使用oneof特性节省内存。oneof字段就像可选字段,除了它们会共享内存,至多一个字段会被设置。设置其中一个字段会清除其它oneof字段。为了在.proto定义oneof字段,你需要在名字前面加上oneof关键字。可以增加任意类型的字段,但是不能使用required、optional、repeated关键字。
可以为.proto文件新增一个可选的package声明符,用来防止不同的消息类型有命名冲突。包的声明符会根据使用语言的不同影响生成的代码。
下面举一个在Caffe中使用的例子:
caffe_tmp.proto内容如下,此proto文件并无实际意义,只是从caffe.proto中选取的一段,为以后分析caffe.proto做个准备:
// syntax关键字,以指明proto文件的Protobuf协议版本,不指明则是v2
// syntax = "proto3"; //v3
syntax = "proto2";
package caffe_tmp;
// Specifies the shape (dimensions) of a Blob.
message BlobShape {
repeated int64 dim = 1 [packed = true];
}
message BlobProto {
optional BlobShape shape = 7;
repeated float data = 5 [packed = true];
repeated float diff = 6 [packed = true];
// 4D dimensions -- deprecated. Use "shape" instead.
optional int32 num = 1 [default = 0];
optional int32 channels = 2 [default = 0];
optional int32 height = 3 [default = 0];
optional int32 width = 4 [default = 0];
}
message Datum {
optional int32 channels = 1;
optional int32 height = 2;
optional int32 width = 3;
// the actual image data, in bytes
optional bytes data = 4;
optional int32 label = 5;
// Optionally, the datum could also hold float data.
repeated float float_data = 6;
// If true data contains an encoded image that need to be decoded
optional bool encoded = 7 [default = false];
}
enum Phase {
TRAIN = 0;
TEST = 1;
}
message NetState {
optional Phase phase = 1 [default = TEST];
optional int32 level = 2 [default = 0];
repeated string stage = 3;
}
// Update the next available ID when you add a new LayerParameter field.
// LayerParameter next available layer-specific
message LayerParameter {
optional string name = 1; // the layer name
optional string type = 2; // the layer type
repeated string bottom = 3; // the name of each bottom blob
repeated string top = 4; // the name of each top blob
// The train / test phase for computation.
optional Phase phase = 10;
// The blobs containing the numeric parameters of the layer.
repeated BlobProto blobs = 7;
}
// Message that stores parameters shared by loss layers
message LossParameter {
// If specified, ignore instances with the given label.
optional int32 ignore_label = 1;
// If true, normalize each batch across all instances (including spatial
// dimesions, but not ignored instances); else, divide by batch size only.
optional bool normalize = 2 [default = true];
}
message ConvolutionParameter {
optional uint32 num_output = 1; // The number of outputs for the layer
optional bool bias_term = 2 [default = true]; // whether to have bias terms
// Pad, kernel size, and stride are all given as a single value for equal
// dimensions in height and width or as Y, X pairs.
optional uint32 pad = 3 [default = 0]; // The padding size (equal in Y, X)
optional uint32 pad_h = 6 [default = 0]; // The padding height
optional uint32 pad_w = 7 [default = 0]; // The padding width
optional uint32 kernel_size = 4; // The kernel size (square)
optional uint32 kernel_h = 8; // The kernel height
optional uint32 kernel_w = 9; // The kernel width
optional uint32 group = 5 [default = 1]; // The group size for group conv
enum Engine {
DEFAULT = 0;
CAFFE = 1;
CUDNN = 2;
}
optional Engine engine = 15 [default = DEFAULT];
}
message MemoryDataParameter {
optional uint32 batch_size = 1;
optional uint32 channels = 2;
optional uint32 height = 3;
optional uint32 width = 4;
}
message PoolingParameter {
enum PoolMethod {
MAX = 0;
AVE = 1;
STOCHASTIC = 2;
}
optional PoolMethod pool = 1 [default = MAX]; // The pooling method
// Pad, kernel size, and stride are all given as a single value for equal
// dimensions in height and width or as Y, X pairs.
optional uint32 pad = 4 [default = 0]; // The padding size (equal in Y, X)
optional uint32 pad_h = 9 [default = 0]; // The padding height
optional uint32 pad_w = 10 [default = 0]; // The padding width
optional uint32 kernel_size = 2; // The kernel size (square)
optional uint32 kernel_h = 5; // The kernel height
optional uint32 kernel_w = 6; // The kernel width
optional uint32 stride = 3 [default = 1]; // The stride (equal in Y, X)
optional uint32 stride_h = 7; // The stride height
optional uint32 stride_w = 8; // The stride width
enum Engine {
DEFAULT = 0;
CAFFE = 1;
CUDNN = 2;
}
optional Engine engine = 11 [default = DEFAULT];
// If global_pooling then it will pool over the size of the bottom by doing
// kernel_h = bottom->height and kernel_w = bottom->width
optional bool global_pooling = 12 [default = false];
}
生成caffe_tmp.pb.h、caffe_tmp.pb.cc文件:protoc.execaffe_tmp.proto --cpp_out=./
分析caffe_tmp.pb.h文件:
因为caffe_tmp.proto中有9个message,因此会产生9个类,按照caffe_tmp.proto从上往下的顺序分别为BlobShape、BlobProto、Datum、NetState、LayerParameter、LossParameter、ConvolutionParameter、MemoryDataParameter、PoolingParameter。这9个类全部在命名空间caffe_tmp内。这9个类全部继承类::google::protobuf::Message。
caffe_tmp.proto中定义了4个枚举,Phase、ConvolutionParameter::Engine、PoolingParameter::PoolMethod、PoolingParameter::Engine。在caffe_tmp.pb.h中会定义这4个枚举,并分别会增加后缀为IsValid、Parse两个返回值为bool的函数,如ConvlutionParameter_Engine_IsValid、ConvolutionParameter_Engine_Parse。
新建一个控制台工程,测试代码如下:
#include "stdafx.h"
#include <iostream>
#include <string>
#include <assert.h>
#include <fstream>
#include "caffe_tmp.pb.h"
int main()
{
// 序列化,将数据存入文件
caffe_tmp::BlobShape blobShape;
blobShape.add_dim(4);
blobShape.add_dim(8);
int size_blobShape = blobShape.ByteSize();
caffe_tmp::BlobProto blobProto;
blobProto.add_data(1.5);
blobProto.add_diff(3.0);
blobProto.set_channels(3);
blobProto.set_height(100);
blobProto.set_width(200);
blobProto.set_num(5);
blobProto.set_data(0, -1.0);
blobProto.add_data(3.3);
caffe_tmp::BlobShape* blobShape1 = blobProto.mutable_shape();
blobShape1->add_dim(10);
int size_blobProto = blobProto.ByteSize();
caffe_tmp::Datum datum;
byte tmp1[5] = { 1, 2, 3, 4, 5 };
datum.set_data(tmp1, 5);
int size_datum = datum.ByteSize();
caffe_tmp::NetState netState;
std::string str[3] = { "hello", "protobuf", "caffe" };
netState.add_stage(str[0]);
netState.add_stage(str[1]);
netState.add_stage(str[2]);
caffe_tmp::Phase phase = caffe_tmp::Phase::TRAIN;
netState.set_phase(phase);
int size_netState = netState.ByteSize();
caffe_tmp::LossParameter lossParameter;
lossParameter.set_normalize(false);
int size_lossParameter = lossParameter.ByteSize();
caffe_tmp::ConvolutionParameter convolutionParameter;
convolutionParameter.set_num_output(2);
convolutionParameter.set_engine (caffe_tmp::ConvolutionParameter::Engine::ConvolutionParameter_Engine_CAFFE);
int size_convolutionParameter = convolutionParameter.ByteSize();
caffe_tmp::PoolingParameter poolingParameter;
poolingParameter.set_pool (caffe_tmp::PoolingParameter::PoolMethod::PoolingParameter_PoolMethod_AVE);
poolingParameter.set_engine (caffe_tmp::PoolingParameter::Engine::PoolingParameter_Engine_CUDNN);
int size_poolingParameter = poolingParameter.ByteSize();
std::fstream output;
output.open("./caffe.bin", std::ios::out | std::ios::trunc | std::ios::binary);
if (!output.is_open()) {
std::cout << "write, open file fail" << std::endl;
return -1;
}
if (!blobShape.SerializeToOstream(&output) || !blobProto.SerializeToOstream(&output) || !datum.SerializeToOstream(&output) ||
!netState.SerializeToOstream(&output) || !lossParameter.SerializeToOstream(&output) ||
!convolutionParameter.SerializeToOstream(&output) || !poolingParameter.SerializeToOstream(&output)) {
std::cout << "failed to write" << std::endl;
return -1;
}
output.close();
// 解析(反序列化)
std::fstream input;
input.open("./caffe.bin", std::ios::in | std::ios::binary);
if (!input.is_open()) {
std::cout << "read, open file fail" << std::endl;
return -1;
}
char* buf = new char[1024];
input.read((char*)buf, size_blobShape);
caffe_tmp::BlobShape blobShape_;
blobShape_.ParseFromString((char*)buf);
assert(blobShape_.ByteSize() == size_blobShape);
assert(blobShape_.dim_size() == 2);
assert(blobShape_.dim(0) == 4);
assert(blobShape_.dim(1) == 8);
input.read(buf, size_blobProto);
caffe_tmp::BlobProto blobProto_;
blobProto_.ParseFromArray(buf, size_blobProto);
assert(blobProto_.ByteSize() == size_blobProto);
assert(blobProto_.has_shape() == true);
blobShape_ = blobProto_.shape();
assert(blobShape_.dim(0) == 10);
assert(blobProto_.data_size() == 2);
assert(blobProto_.data(0) == -1.0);
assert(blobProto_.diff(0) == 3.0);
assert(blobProto_.has_num() == true);
input.read(buf, size_datum);
caffe_tmp::Datum datum_;
datum_.ParseFromArray(buf, size_datum);
assert(datum_.ByteSize() == size_datum);
assert(datum_.has_channels() == false);
assert(datum_.float_data_size() == 0);
assert(datum_.has_data() == true);
std::string str1 = datum_.data();
assert(str1.size() == 5);
std::vector<byte> bytes(str1.begin(), str1.end());
assert(bytes[4] == 5);
input.read(buf, size_netState);
caffe_tmp::NetState netState_;
netState_.ParseFromArray(buf, size_netState);
assert(netState_.ByteSize() == size_netState);
assert(netState_.has_phase() == true);
caffe_tmp::Phase phase_ = netState_.phase();
assert(phase_ == 0);
assert(netState_.has_level() == false);
assert(netState_.stage_size() == 3);
assert(netState_.stage(1) == "protobuf");
input.read(buf, size_lossParameter);
caffe_tmp::LossParameter lossParameter_;
lossParameter_.ParseFromArray(buf, size_lossParameter);
assert(lossParameter_.ByteSize() == size_lossParameter);
assert(lossParameter_.has_ignore_label() == false);
assert(lossParameter_.normalize() == false);
input.read(buf, size_convolutionParameter);
caffe_tmp::ConvolutionParameter convolutionParameter_;
convolutionParameter_.ParseFromArray(buf, size_convolutionParameter);
assert(convolutionParameter_.ByteSize() == size_convolutionParameter);
assert(convolutionParameter_.has_kernel_size() == false);
assert(convolutionParameter_.num_output() == 2);
assert(convolutionParameter_.has_engine() == true);
caffe_tmp::ConvolutionParameter_Engine engine_ = convolutionParameter_.engine();
assert(engine_ == caffe_tmp::ConvolutionParameter::Engine::ConvolutionParameter_Engine_CAFFE);
input.read(buf, size_poolingParameter);
caffe_tmp::PoolingParameter poolingParameter_;
poolingParameter_.ParseFromArray(buf, size_poolingParameter);
assert(poolingParameter_.ByteSize() == size_poolingParameter);
assert(poolingParameter_.has_pool() == true);
caffe_tmp::PoolingParameter_PoolMethod poolMethod = poolingParameter_.pool();
assert(poolMethod == caffe_tmp::PoolingParameter::PoolMethod::PoolingParameter_PoolMethod_AVE);
caffe_tmp::PoolingParameter_Engine pooling_engine_ = poolingParameter_.engine();
assert(pooling_engine_ == 2);
delete[] buf;
input.close();
return 0;
}